Scaling Test-Time Compute Without Verification or RL is Suboptimal
Best AI papers explained - A podcast by Enoch H. Kang
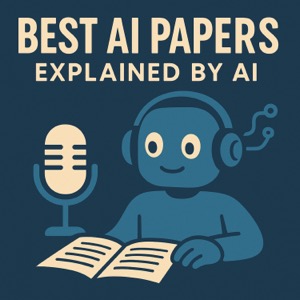
Categories:
The paper presents a theoretical analysis comparing verifier-based (VB) and verifier-free (VF) algorithms for training large language models (LLMs) under varying compute budgets.It demonstrates that VB methods outperform VF methods as test-time compute increases, particularly when the base LLM exhibits high heterogeneity and anti-concentration in reward distributions.The findings indicate that while both methods can be effective, VB methods scale better with larger budgets, and this gap widens with more prompts for finetuning.Empirical results support the theoretical claims, showing that common pre-trained LLMs often meet the necessary conditions for VB advantages