Test-Time RL: Self-Evolving LLMs via Majority Voting Rewards
Best AI papers explained - A podcast by Enoch H. Kang
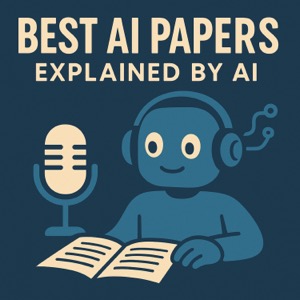
Categories:
This paper introduces Test-Time Reinforcement Learning (TTRL), a novel method for enhancing large language models by applying reinforcement learning on unlabeled test data. TTRL tackles the challenge of reward estimation without ground truth by using majority voting among multiple model-generated responses as a proxy for correct answers, which then guides the RL training process. Experiments demonstrate that TTRL significantly improves performance across various reasoning tasks and models, often surpassing the initial capabilities and approaching the results of models trained with labeled data. This approach highlights a promising direction for self-evolution and continual learning in LLMs without reliance on extensive human annotation